top of page
Our Projects
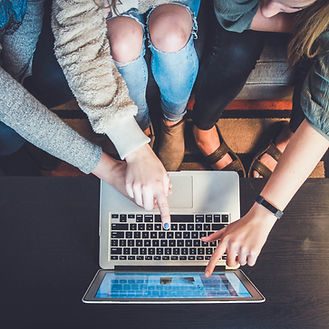
NSF
ProtoOKN Theme 3: EduGate
Prototype-Open Knowledge Network (OKN) project seeks to ensuring the growth of a community of learning and practice centered on the OKN and its use in solving critical, real-world problems. Proto-OKN Education Gateway, EduGate, effort will provide a comprehensive education platform that would also facilitate close collaborations and convergence among current OKN projects, as well as future entrants to the network.
bottom of page